
OUR METHODOLOGY: THE DATA SCIENCE PYRAMID
The Data Science Pyramid emphasizes the strong data foundation that is required to reach full data science maturity. The pyramid begins with the raw data itself, which may come from a variety of sources, in various formats, and in vast amounts. Data Engineering establishes the context and structure that is required for data to become information. Master Data Management and Governance builds upon this premise to insure coordination and quality before the data passes into the final phases. Reporting and Business Intelligence represents the beginning of insight gathering, where information is aggregated, sliced and diced to facilitate analysis. Finally, Data Science represents the culmination of data into action, building upon each of the foundations while also bringing in a new set of powerful statistical approaches.
The data science pyramid is not necessarily linear, meaning that an organization does not need to be perfect in each layer before moving onto the next. Rather, a certain level of maturity is required in each before advancing, and each consecutive rise in level informs improvements to those below. For example, a company could, having established a grasp on its Master Data Management and Governance, move onto Reporting and Business Intelligence, only to find further areas for improvement around data quality. The data science pyramid is indicative of value potential; if an organization has not already established a strong data foundation, then it is not wise to jump levels in most instances. Rather, organizations would likely unlock more initial value by focusing on the fundamentals before moving onto data science maturity. A statistical model is usually only as good as the information it is trained on, which is in turn the direct result of the underlying sources, infrastructure, governance and reporting.
-
Data Acquisition focuses on the various sources of raw data, which can range from more traditional BAU sources such as ERP systems, Legacy Data Stores, and Operational Systems to more exotic ones such as social media sites and natural language. Data science has broadened what is possible in data acquisition, as previously un-analyzable types of data can now be made useful through advanced techniques.
-
Data Engineering is all of the activities associated with processing, moving and storing data. Data Engineering can range from traditional tool-based ETL to custom developed data pipelines, all of which create the underlying infrastructure through which data flows and sits. It is vital to data science, because it provides the tools and processes necessary for the ETL workflows that allow data to move efficiently and be leveraged for advanced uses further up the pyramid.
-
Master Data Management and Governance requires that intense scrutiny be placed on the meta-attributes of data. From data types to cardinality to value distribution, this umbrella term captures the various activities associated with improving the quality and usability of data through clean up and feature enrichment. MDM is a crucial middle component because the algorithms that drive AI and Machine Learning consume, analyze, and learn from data. Therefore, data must be accurate, up-to-date, and plentiful.
-
Reporting and Business Intelligence encompasses the tools and techniques associated with making information readily available to organizations for analytical judgement. The emphasis lies on presenting information in a compelling and consumable manner for the use of human decision making, and commonly incorporates elements of data visualization and OLAP data schemas. Reporting and BI add value because it allows effective communication of your data science outputs and results to the rest of the organization. It is the bridge that connects data science to the key decision makers who can then make informed and data-driven decisions that maximize the benefit of the company.
-
Data Science exists at the intersection of advanced mathematical, computer science, and domain expertise. It is an interdisciplinary approach to drawing diagnostic, predictive, or prescriptive insights out of large, complex, and/or exotic data sources using formal, rigorous, and reproducible methods.
The entire concept of the pyramid gets to the core of why and how we use data. In our efforts to turn data into information into insight, we have built massive IT systems to turn raw 1s and 0s into action items. Every step you go up the pyramid, you are automating/improving some portion of the data/information/insight process; for example, data infrastructure & engineering turns the raw information into something with more context/structure (and so on). The jump from Reporting & BI to Data Science represents the last step of this automation. We are trying to automate the insight gathering work of the analytical consumers of BI reports, and to ultimately automate the decisions themselves.
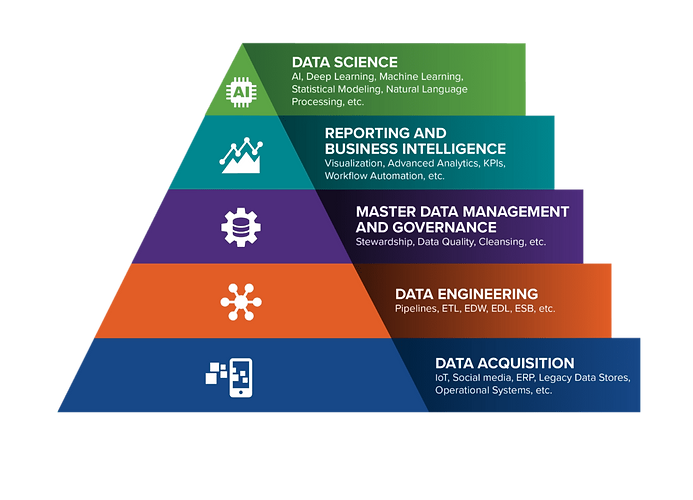